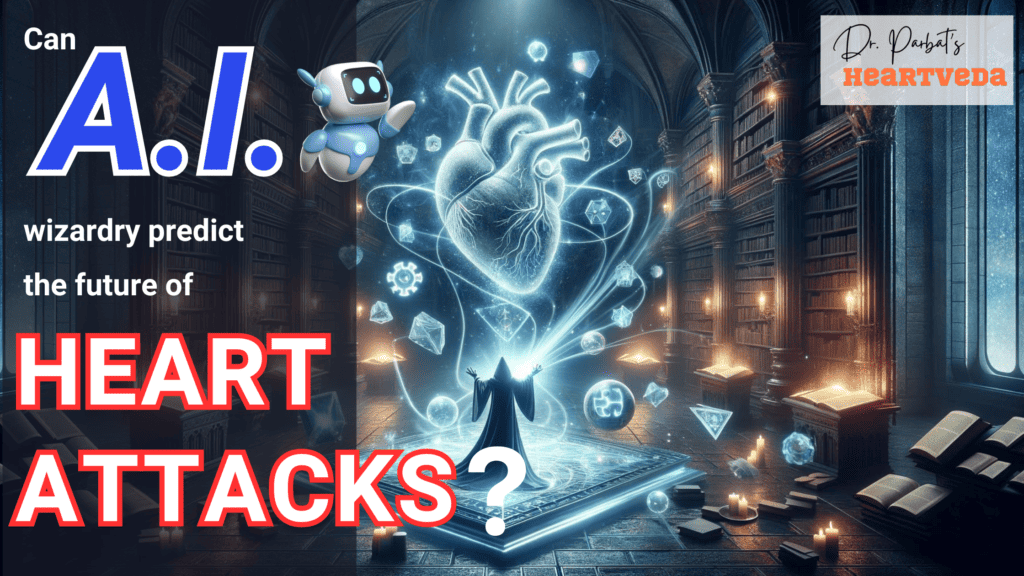
Introduction
Can a machine tell if your heart is at risk? 🤖💔 Imagine if a crystal ball could peek into the future of your heart’s health, offering a glimpse of what lies ahead. For busy Indian professionals in their 30s, 40s, and 50s, this isn’t just a flight of fancy but a reality brought closer by artificial intelligence (AI). This blog unveils the groundbreaking strides AI has made in predicting heart disease risk, potentially transforming the landscape of cardiovascular care.
With a study revealing AI’s capability to forecast heart attack risks up to a decade in advance, the implications are profound. Analyzing data from over 40,000 individuals, AI tools are now able to pinpoint those at high risk of cardiac events with astonishing precision. This blog will guide you through the ins and outs of how AI and machine learning algorithms are becoming the new guardians of heart health, offering hope and actionable insights to those at risk.
From the mechanics of AI technology in heart disease prediction to the benefits it brings to early detection and prevention, we’re on the brink of a healthcare revolution. This friendly and motivating read is designed to simplify complex information into easy-to-understand insights, ensuring you’re well-informed about the potential AI holds in safeguarding your heart’s future. Let’s embark on this journey of discovery together, exploring how AI is setting the stage for a healthier tomorrow. 🚀❤️
AI for the Heart
Part -1
In the sprawling urban landscape of Mumbai, where the future is built on the foundations of technology and innovation, lived Vandana, a dedicated AI researcher. Her life was a testament to the power of artificial intelligence, with days spent developing algorithms that could potentially transform lives. Vandana, a mother of one and a beacon of inspiration in the tech community, believed in harnessing AI for the greater good, yet she seldom applied this revolutionary technology to her personal life.
Despite her deep understanding of AI’s capabilities and her emotional intelligence in navigating the complexities of technology, Vandana’s approach to her health was surprisingly traditional. She lived in a mental frame that considered health check-ups and lifestyle changes only after symptoms appeared, not before.
AI Technology in Predicting Heart Attack
Artificial intelligence (AI) technology is revolutionizing the field of cardiovascular health by enabling the prediction of heart attack risk with unprecedented accuracy. A groundbreaking study led by Professor Charalambos Antoniades at the University of Oxford has harnessed the power of AI to develop a tool capable of independently and precisely predicting the risk of cardiac events.
The AI tool incorporates machine learning algorithms and leverages data on various clinical risk factors, including changes in the fat surrounding inflamed arteries and arterial narrowings. By analyzing this data, the AI tool can identify high-risk individuals and provide early indications of an imminent heart attack.
This AI technology holds great promise in enhancing early identification and preventive treatment strategies for individuals at high risk for heart attacks. By detecting potential cardiac events well in advance, healthcare professionals can implement timely interventions such as lifestyle modifications and medication therapies to reduce the risk and severity of heart attacks.
Incorporating AI technology in predicting heart attack risk signifies a major advancement in cardiovascular healthcare. By harnessing the power of machine learning and data analysis, AI tools can exponentially improve the accuracy and efficiency of heart attack prediction, enabling proactive measures to save lives and improve patient outcomes.
In Professor Antoniades’ own words, “AI technology provides us with the ability to analyze complex data and identify subtle patterns that humans may overlook. This transformative breakthrough has immense potential to revolutionize the way we predict, prevent, and manage heart attacks.”
Benefits of AI Technology in Predicting Heart Attack | |
Improved early identification of high-risk individuals | |
Precise assessment of cardiac event risk | |
Implementation of timely interventions and preventive measures | |
Enhanced accuracy and efficiency in heart attack prediction | |
Revolutionizing cardiovascular healthcare |
Traditional Methods of Heart Attack Prediction
When it comes to predicting the likelihood of a heart attack, physicians have traditionally relied on standard assessments that take into account various factors. These methods have proven to be effective to some extent in assessing the risk of heart disease. Let’s take a closer look at the traditional methods used for heart attack prediction.
1. Cholesterol Levels
One of the key factors considered in heart attack prediction is cholesterol levels. High levels of LDL cholesterol (commonly known as bad cholesterol) can lead to the formation of plaque in the arteries, increasing the risk of heart disease. Monitoring cholesterol levels is crucial in assessing an individual’s risk of a heart attack.
2. Blood Pressure
Another important factor in heart attack prediction is blood pressure. High blood pressure, or hypertension, can damage the arteries and make them more susceptible to blockages. Monitoring blood pressure regularly helps in identifying individuals at risk of heart disease.
3. Lifestyle Factors
Lifestyle choices play a significant role in heart attack prediction. Factors such as smoking, lack of physical activity, and an unhealthy diet contribute to an increased risk of heart disease. Assessing an individual’s lifestyle choices helps in identifying those at higher risk.
4. Health Conditions
Existing health conditions can also contribute to the prediction of heart attacks. Conditions such as diabetes, obesity, and chronic kidney disease can increase the risk of cardiovascular problems. Evaluating an individual’s health conditions provides valuable insights into their heart attack risk.
While these traditional methods have been widely used and proven effective to some extent, new technologies are being developed to improve the accuracy and early prediction of heart attacks. These advancements aim to enhance the assessment of heart attack risks and enable early intervention, ultimately saving lives and improving patient outcomes.
Comparison of Traditional Heart Attack Prediction Methods
Methods | Key Factors Assessed | Advantages | Limitations |
Cholesterol Levels | LDL cholesterol (bad cholesterol) | Easy to measure, widely available | Doesn’t consider other risk factors |
Blood Pressure | Blood pressure readings | Simple, non-invasive measurement | May not capture long-term fluctuations |
Lifestyle Factors | Smoking, physical activity, diet | Reflects individual’s overall health | Relies on self-reporting, subjectivity |
Health Conditions | Diabetes, obesity, kidney disease | Considers specific risk factors | May not account for genetic factors |
AI and Face-to-face Prediction
When it comes to predicting heart attacks, the combination of artificial intelligence (AI) and face-to-face interaction with doctors is proving to be a game-changing approach. Dr. Stephen Weng and his team at the University of Nottingham have developed an innovative algorithm that harnesses the power of AI to predict heart attacks with remarkable accuracy.
The AI algorithm, which has been trained using electronic patient records, can analyze specific data and identify patterns that suggest an imminent heart attack. By combining this AI analysis with face-to-face interaction between doctors and patients, healthcare professionals are empowered to intervene early with appropriate treatments and lifestyle changes. This proactive approach helps to reduce the risk of heart attack and improve overall cardiovascular health.
“By utilizing the advanced capabilities of AI, we can now predict heart attacks up to 10 years in advance, with an impressive 76 percent accuracy,” says Dr. Biprajit Parbat. “This breakthrough technology has the potential to revolutionize the way we approach heart disease prevention and treatment.”
The combination of AI and face-to-face prediction offers a personalized and proactive approach to healthcare. By harnessing the power of AI algorithms, doctors can better assess individual risk factors and tailor treatment plans accordingly. This not only improves patient outcomes but also reduces the burden on healthcare systems.
It’s important to note that AI algorithms are not meant to replace doctors but rather enhance their abilities. The analysis provided by AI algorithms serves as a valuable tool for healthcare professionals, allowing them to make informed decisions based on comprehensive data and insights. Ultimately, this collaborative approach between AI and doctors leads to more accurate predictions, more effective treatments, and better patient outcomes.
Advantages of AI and Face-to-face Prediction:
- Early detection of heart attack risk
- Personalized treatment plans
- Improved patient outcomes
- Reduced burden on healthcare systems
This remarkable advancement in heart attack prediction showcases the potential of AI technology to revolutionize the field of healthcare. By combining the strengths of AI algorithms and face-to-face interaction, we are one step closer to a future where heart attacks can be accurately predicted and prevented.
AI for the Heart
Part – 2
The turning point in Vandana’s life came unexpectedly during a tech conference where she presented her latest project on AI’s predictive power in healthcare. After her presentation, a renowned cardiologist approached her, intrigued by the potential of her work. He explained how AI could predict the risk of heart attacks up to a decade in advance, emphasizing the importance of such technology in preventive healthcare.
Vandana’s darkest moment wasn’t a health scare of her own but the realization that her father, who had recently suffered a minor heart attack, could have benefited from the very technology she was developing. This revelation struck a chord, highlighting a gap between her professional achievements and personal applications of her work.
Motivated by this insight and guided by the cardiologist’s expertise, Vandana embarked on a mission to bridge this gap. She began to work closely with healthcare professionals, integrating AI algorithms with medical data to develop a predictive tool for heart health. This tool analyzed various factors, including genetics, lifestyle, and environmental influences, offering individuals a personalized risk assessment and recommendations for preventive measures.
Predicting Heart Attack Through Retinal Scans
Recent research conducted by Dr. Lily Peng and her team at Google has opened up new possibilities for predicting heart attacks using retinal scans. The team is exploring how blood vessels in the eyes can serve as indicators of heart attack risk. By analyzing retinal scans and incorporating the data into an AI algorithm, they have made significant progress in identifying patients who have experienced cardiovascular events.
Retinal scans provide valuable information about the blood vessels in the eyes, which can reflect the overall health of the cardiovascular system. The presence of certain abnormalities or changes in these blood vessels can indicate an increased risk of heart attack.
Early testing of this innovative approach has shown promise in accurately predicting heart attack risk. As the technology continues to advance, retinal cameras may become a standard, non-invasive tool for assessing heart health and identifying individuals who are at heightened risk of experiencing a heart attack.
Advantages of Predicting Heart Attack Through Retinal Scans | Challenges of Predicting Heart Attack Through Retinal Scans |
Non-invasive procedure | Access to retinal scanning equipment |
Scalable and cost-effective | Interpreting and analyzing retinal images accurately |
Potential for early detection and intervention | Evaluating the effectiveness of retinal scans in diverse populations |
Overall, predicting heart attacks through retinal scans holds great promise in improving the early detection and prevention of cardiovascular events. As further advancements and research are made in this field, this innovative approach could save countless lives and significantly enhance overall cardiac care.
Quantifying Plaque for Heart Attack Prediction

Researchers at Cedars-Sinai have developed an AI-enabled tool that revolutionizes the prediction of heart attacks by accurately quantifying plaque buildup in arteries. This groundbreaking technology uses a coronary computed tomography angiography (CTA) to generate highly detailed 3D images of the heart and arteries, allowing doctors to assess the extent of plaque and artery narrowing.
The AI algorithm embedded in the tool analyzes the CTA images in just a few seconds, precisely measuring the amount of plaque present. This quantitative assessment provides valuable information for predicting the risk of a heart attack, enabling healthcare professionals to intervene early and implement appropriate preventive measures.
By accurately quantifying plaque, this AI-powered tool enhances the accuracy of heart attack prediction, improving patient outcomes and potentially saving lives. Let’s take a closer look at how the process works:
- The patient undergoes a coronary computed tomography angiography (CTA) scan, a non-invasive imaging procedure.
- The CTA scan captures detailed 3D images of the heart and arteries, highlighting plaque buildup and artery narrowing.
- The AI algorithm analyzes the CTA images, quantifying the amount of plaque present.
- Based on the plaque measurement, the tool predicts the likelihood of a heart attack.
- Healthcare professionals can then intervene early and implement appropriate treatments, lifestyle changes, and preventive measures.
This AI-enabled tool not only revolutionizes heart attack prediction but also paves the way for personalized healthcare, enabling tailored interventions and preventive strategies based on each individual’s unique risk profile.
Now, let’s take a closer look at the quantitative assessment of plaque buildup:
Plaque Level | Risk of Heart Attack |
Low | Minimal risk |
Moderate | Moderate risk |
High | Significant risk |
Severe | High risk |
The table above illustrates the correlation between different plaque levels and the associated risk of a heart attack. By accurately measuring and quantifying plaque buildup, healthcare professionals can assess the risk level for each patient and make informed decisions regarding their treatment and preventive measures.
With the advent of this AI-powered tool for quantifying plaque, medical professionals can now predict the likelihood of a heart attack with greater accuracy, resulting in early intervention and improved patient outcomes. This cutting-edge technology represents a significant advancement in the field of heart disease prevention and treatment.
Measurement | Agreement with Experts (ICC) | Agreement with Ultrasound (ICC) | Analysis Time (Deep Learning vs Experts) | Outcome Over 4.7 Years | Significant Plaque Volume for Heart Attack Risk | Adjusted Hazard Ratio for Heart Attack |
---|---|---|---|---|---|---|
Total Plaque Volume | 0.964 | 0.949 | 5.65 seconds vs 25.66 minutes | Not Directly Measured | 238.5 mm³ or higher | 5.36 |
Percent Diameter Stenosis | 0.879 | Not Applicable | Not Applicable | Not Directly Measured | Not Applicable | 2.49 (for obstructive stenosis) |
Minimal Luminal Area | Not Applicable | 0.904 | Not Applicable | Myocardial Infarction in 2.5% of Patients | Not Applicable | 1.01 (for ASSIGN score) |
This table shows that AI has excellent agreement with both expert assessments and ultrasound measurements for detecting heart attack risk, especially in measuring total plaque volume. It highlights the efficiency of AI in analyzing data quickly and its ability to predict heart attack risk based on plaque volume.
Combining Biomarkers and Gut Bacteria Analysis
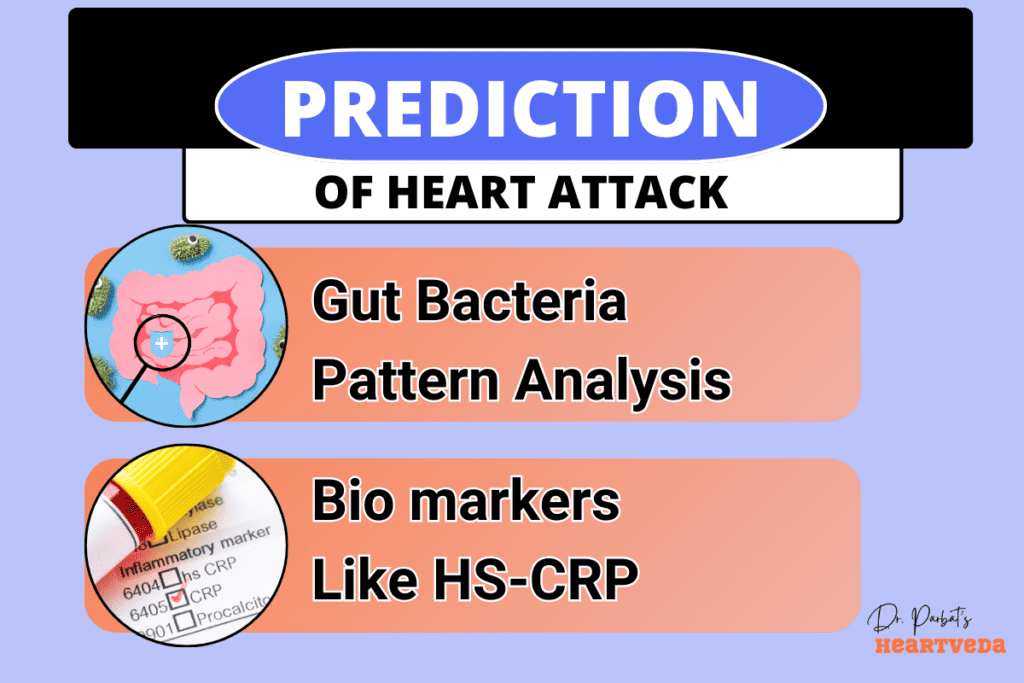
Cardiologists are studying the potential of combining biomarkers and analysis of gut bacteria in predicting the likelihood of a heart attack. By measuring levels of molecules like trimethylamine N-oxide (TMAO), which is produced by gut bacteria, and analyzing specific biomarkers, doctors can gain valuable insights into a patient’s risk of heart problems. Incorporating these additional factors into existing predictive methods may enhance the accuracy of heart attack prediction.
Gut Bacteria and Heart Health
Gut bacteria play a crucial role in our overall health, and emerging research suggests a connection between the composition of gut bacteria and heart disease. Certain bacteria in the gut produce molecules that can affect heart health, such as TMAO. Increased levels of TMAO have been associated with an elevated risk of developing cardiovascular problems, including heart attacks.
Scientists have observed that the abundance and diversity of gut bacteria can vary among individuals, and these differences may impact their susceptibility to heart disease. By analyzing the composition and activity of gut bacteria, researchers aim to identify patterns that can help predict an individual’s risk of experiencing a heart attack.
Biomarkers for Heart Attack Prediction
In addition to gut bacteria analysis, biomarkers are another vital tool in predicting heart attacks. Biomarkers are measurable substances in the body that can indicate an increased risk of a particular condition. In the case of heart attacks, certain biomarkers have been identified as indicators of cardiovascular disease and potential cardiac events.
Examples of biomarkers used in heart attack prediction include elevated levels of C-reactive protein (CRP), which indicates inflammation in the body, and high levels of troponin, a protein released during heart muscle damage. When combined with other risk factors and predictive methods, analyzing these biomarkers can provide valuable information for assessing an individual’s likelihood of experiencing a heart attack.
The Potential of Combined Analysis
By combining gut bacteria analysis and biomarker evaluation, cardiologists can gain a more comprehensive understanding of an individual’s heart attack risk. This multifaceted approach allows doctors to consider multiple factors that contribute to the development of cardiovascular disease.
“The combination of analyzing gut bacteria and biomarkers provides a more complete picture of a patient’s heart health and helps us make more accurate predictions about their risk of heart attack. This knowledge enables us to tailor preventive strategies and interventions to reduce that risk.” – Dr. Biprajit Parbat
Furthermore, the integration of gut bacteria analysis and biomarker evaluation with other predictive methods, such as AI algorithms and imaging techniques, may lead to even more precise and personalized heart attack prediction.
The Future of Heart Attack Prediction
As ongoing research continues to uncover the intricacies of the gut-heart connection and explore additional biomarkers, the field of heart attack prediction holds immense promise. The combination of gut bacteria analysis and biomarker evaluation has the potential to significantly enhance our ability to identify individuals at high risk of experiencing a heart attack.
As this field progresses, it is essential to ensure that these predictive methods are accessible and widely available to individuals around the world. By integrating these advancements into routine healthcare, we can empower individuals to take preventative measures and make informed choices to reduce their risk of heart disease.
AI for the Heart
Part – 3
Vandana’s journey of innovation was not just a professional endeavor but a deeply personal one. She became a pioneer in the field of AI-driven preventive healthcare, advocating for the early adoption of technology to predict and prevent heart attacks. Her work inspired a shift in how people approached their health, emphasizing prevention over treatment.
As Vandana’s technology gained recognition, it transformed the lives of many, including her own family. She ensured her father was among the first to benefit from her AI tool, which recommended specific lifestyle changes and regular monitoring to manage his heart health effectively.
The Promise and Future of Early Heart Attack Prediction
The advancements in AI, retinal scans, quantifying plaque, and analyzing biomarkers offer promising possibilities for the future of heart attack prediction. These technologies, when combined with traditional methods and clinical assessments, have the potential to revolutionize the way heart disease is diagnosed and treated.
Artificial intelligence has already shown great potential in predicting an individual’s risk of a heart attack up to a decade in advance. Through the use of AI algorithms, researchers have been able to analyze large sets of data and identify patterns and risk factors that were previously difficult to detect manually. This can lead to early intervention and preventative measures that can drastically reduce the chances of a heart attack.
Retinal scans provide a non-invasive method of predicting heart attack risk. By analyzing the blood vessels in the eyes, AI algorithms can identify patients who have experienced a cardiovascular event and determine their risk of future heart problems. This technology has the potential to become a standard test for heart patients, allowing for early detection and treatment.
Quantifying plaque buildup in arteries is crucial for predicting the likelihood of a heart attack. AI-enabled tools, such as coronary computed tomography angiography (CTA) imaging, can accurately measure and assess the extent of plaque and artery narrowing. This information can then be used to predict an individual’s risk and guide preventative measures and treatment plans.
The analysis of biomarkers and gut bacteria can also provide valuable insights into a patient’s likelihood of a heart attack. Certain molecules produced by gut bacteria, such as trimethylamine N-oxide (TMAO), have been linked to heart problems. By measuring these molecules and analyzing other biomarkers, healthcare professionals can better assess a patient’s risk and tailor preventive strategies accordingly.
The future of heart attack prediction holds great promise. Through the continued advancements in AI, retinal scans, quantifying plaque, and analyzing biomarkers, we are moving towards a future where heart attacks can be accurately predicted and prevented. These technologies, combined with traditional methods, have the potential to save countless lives and significantly improve outcomes for patients with heart disease.
Advancements | Potential Benefits |
AI in predicting heart attack | Early identification of high-risk individuals |
Retinal scans | Non-invasive, early detection of cardiovascular events |
Quantifying plaque buildup | Accurate prediction of heart attack risk |
Analysis of biomarkers and gut bacteria | Improved assessment of heart attack risk |
The Importance of Early Intervention and Prevention
Early intervention and prevention are crucial in reducing the risk of heart attacks. By utilizing predictive technologies and strategies, healthcare professionals can identify high-risk individuals and implement appropriate treatments to manage cholesterol, blood pressure, and lifestyle factors. This proactive approach can help individuals reduce their risk and improve overall cardiovascular health.
AI for the Heart
END
Vandana’s story is a powerful reminder of the potential of artificial intelligence to revolutionize healthcare, predicting risks and empowering individuals to take control of their health long before symptoms appear. It challenges us to think about how we can apply cutting-edge technology to not just advance our careers but to enhance our well-being and that of those around us.
Vandana’s narrative prompts us to ask: Are we fully leveraging the technology at our fingertips to safeguard our future, or are we waiting for a wake-up call to recognize its value in our lives?
Conclusion
In conclusion, the recent advances in heart attack prediction have revolutionized the field of healthcare. The integration of artificial intelligence (AI) technology, retinal scans, quantifying plaque, and analyzing biomarkers has shown great promise in early detection and intervention. By combining these innovative methods with traditional approaches, healthcare professionals have the potential to save countless lives and improve outcomes for patients with heart disease.
The future of heart attack prediction looks promising, with ongoing research and development aimed at further enhancing the accuracy and effectiveness of these techniques. Continued advancements in AI algorithms, retinal scanning technology, plaque quantification, and biomarker analysis will pave the way for a future where heart attacks can be accurately predicted and even prevented. This will not only transform the way heart disease is diagnosed and treated but also revolutionize cardiovascular healthcare as a whole.
Early intervention and prevention remain key to reducing the risk of heart attacks. By identifying high-risk individuals through predictive technologies, healthcare professionals can implement timely interventions and preventive measures to manage cholesterol, blood pressure, and lifestyle factors. This proactive approach, coupled with the advancements in heart attack prediction, holds the potential to significantly reduce the burden of heart disease and improve overall cardiovascular health.
Key Takeaways:
- AI technology has the potential to predict the risk of heart attacks up to ten years in advance.
- A study funded by the British Heart Foundation demonstrated the effectiveness of AI in predicting cardiac events.
- This technology has the potential to revolutionize the treatment of heart disease and improve patient outcomes.
- The use of AI in predicting heart attacks can help identify high-risk individuals and implement preventive measures.
- Continued research and development in this field hold the promise of eradicating or significantly reducing heart attacks in the future.
Q: What is the importance of predicting heart disease using machine learning algorithms?
A: Predicting heart disease using machine learning algorithms is crucial for early detection and effective intervention, which can potentially save lives. This approach can assist in predicting the occurrence of heart disease and contributing factors, allowing for personalized preventive measures and timely treatment.
Q: How does machine learning technique contribute to the prediction of heart disease?
A: Machine learning techniques play a significant role in predicting heart disease by analyzing large datasets to identify patterns and correlations that may not be easily recognizable through traditional methods. This enables the development of accurate predictive models based on various risk factors and patient data.
Q: Can machine learning models accurately predict cardiovascular disease?
A: Yes, machine learning models have demonstrated the capability to accurately predict cardiovascular disease by leveraging complex algorithms that can analyze diverse data sources, such as medical history, lifestyle factors, and diagnostic tests, to generate precise predictions and risk assessments.
Q: What are some common machine learning algorithms used for heart disease prediction?
A: Some common machine learning algorithms used for heart disease prediction include decision trees, support vector machines, neural networks, and deep learning approaches. These algorithms are utilized to develop sophisticated models that can effectively predict the occurrence and diagnosis of heart-related conditions.
Q: How is the American Heart Association involved in the prediction of heart disease using machine learning?
A: The American Heart Association is actively involved in supporting research and initiatives related to the application of machine learning techniques for heart disease prediction. They collaborate with researchers and organizations to advance the development of efficient predictive models and data-driven approaches for cardiovascular health.
Q: What role does data mining play in the prediction of heart disease?
A: Data mining plays a crucial role in the prediction of heart disease by extracting valuable insights and patterns from large datasets. This process helps in identifying predictive features and risk factors, contributing to the development of accurate models for disease prediction and early detection.
Q: Why is early detection of heart disease important?
A: Early detection of heart disease is essential as it allows for timely interventions and proactive management of risk factors, potentially preventing the progression of the condition and reducing the likelihood of severe complications. It enables individuals to receive appropriate medical attention and adopt lifestyle changes for better cardiovascular health.
Q: What are the key factors used in the prediction of heart disease using machine learning techniques?
A: The prediction of heart disease using machine learning techniques involves analyzing various factors such as heart rate, blood flow to the heart, medical history, lifestyle habits, diagnostic test results, and the presence of specific risk factors related to coronary heart disease and heart failure.
Q: How can machine learning contribute to effective heart disease prediction using diverse datasets?
A: Machine learning techniques can contribute to effective heart disease prediction by processing and analyzing diverse datasets, including patient health records, genetic information, imaging studies, and lifestyle data. This comprehensive approach enables the development of more accurate and personalized predictive models.
Q: What role does machine learning play in the development of a heart disease prediction system?
A: Machine learning plays a pivotal role in the development of a heart disease prediction system by enabling the creation of advanced algorithms that can assess multiple risk factors, evaluate complex relationships within data, and provide personalized predictions, thereby contributing to the early detection and prevention of cardiovascular diseases.
Q: What is the importance of heart disease prediction using machine learning algorithms?
A: Predicting heart disease using machine learning algorithms is crucial for early diagnosis and timely intervention, which can significantly improve patient outcomes and save lives.
Q: How can machine learning algorithms aid in the diagnosis of heart disease?
A: Machine learning algorithms can analyze large sets of medical data to identify patterns and trends that can be used to accurately diagnose and predict the presence of heart disease in patients.
Q: What are some common machine learning techniques used in predicting heart disease?
A: Common machine learning techniques used for heart disease prediction include decision trees, support vector machines, logistic regression, and neural networks.
Q: Can machine learning algorithms improve the performance of heart disease prediction models?
A: Yes, machine learning algorithms can help improve the performance of heart disease prediction models by effectively identifying relevant features and patterns in the data, leading to more accurate predictions.
Q: How can data mining techniques contribute to heart disease prediction?
A: Data mining techniques can aid in extracting valuable insights from large datasets, which can then be used to train machine learning models for accurate prediction of heart disease.
Q: What role do classification algorithms play in heart disease prediction?
A: Classification algorithms are essential in categorizing patients based on their health data, enabling the prediction of the presence or absence of heart disease using machine learning techniques.
Q: What are some widely used datasets for heart disease prediction in machine learning research?
A: Datasets such as the UCI Machine Learning Repository’s heart disease dataset and other international conference datasets are commonly used for training and testing machine learning models for heart disease prediction.
Q: How do machine learning-based prediction models contribute to the diagnosis of heart disease?
A: Machine learning-based prediction models utilize patient data to provide accurate diagnosis and risk assessment for heart disease, aiding healthcare professionals in making informed decisions.
Q: Can machine learning techniques aid in the early detection of coronary artery disease?
A: Yes, machine learning techniques can aid in the early detection of coronary artery disease by analyzing various patient parameters and identifying potential indicators of the condition.
Q: What are the benefits of using machine learning algorithms for heart disease prediction?
A: The benefits include improved accuracy in diagnosing heart disease, early detection of potential cardiac issues, and the ability to develop personalized treatment plans based on predictive analytics.
Q: What is heart disease prediction using machine learning?
A: Heart disease prediction using machine learning involves using data mining and various machine learning algorithms to develop a system that can accurately diagnose and predict the likelihood of heart disease in patients.
Q: How does machine learning contribute to heart disease prediction?
A: Machine learning techniques enable the analysis of large amounts of data to identify patterns and trends that can be used to predict heart disease. This involves using machine learning classifiers and supervised learning methods to develop accurate prediction models.
Q: What are the benefits of using machine learning algorithms for heart disease prediction?
A: Machine learning algorithms can provide accurate and efficient diagnosis and prediction of heart disease, enabling early detection and intervention, which can improve patient outcomes and reduce healthcare costs.
Q: What are some common machine learning methods used in heart disease prediction?
A: Common machine learning methods used in heart disease prediction include artificial neural networks, classification techniques, and hybrid machine learning approaches that combine multiple algorithms for improved accuracy.
Q: How is data utilized in heart disease prediction using machine learning?
A: Data from various sources, including the UCI Machine Learning Repository and heart rate variability measurements, are used to train and test machine learning models for heart disease prediction. This data is crucial for developing accurate prediction systems.
Q: How accurate are machine learning algorithms in predicting heart disease?
A: Machine learning algorithms have been shown to achieve high levels of accuracy in predicting heart disease, including the ability to detect true positive cases of cardiovascular disease and to classify the likelihood of developing specific heart conditions.
Q: What is the role of machine learning in developing disease prediction systems?
A: Machine learning plays a crucial role in developing disease prediction systems by providing the capability to analyze and interpret diverse data sets, leading to improved accuracy in predicting and diagnosing heart disease and other health conditions.
Q: Can machine learning techniques assist in detecting coronary heart disease?
A: Yes, machine learning techniques can assist in detecting coronary heart disease by analyzing patient data and identifying patterns indicative of the condition, leading to earlier diagnosis and intervention.
Q: How can machine learning facilitate the prediction of congestive heart failure?
A: Machine learning algorithms can facilitate the prediction of congestive heart failure by analyzing relevant patient data and identifying indicators of developing heart disease, enabling proactive management and treatment.
Q: Are there any specific machine learning approaches for developing heart disease prediction systems?
A: Yes, hybrid machine learning approaches that combine various algorithms and techniques are increasingly being used for developing heart disease prediction systems, leading to enhanced prediction accuracy and reliability.