Did you know that data mining techniques are playing a crucial role in predicting heart disease? In the healthcare industry, vast amounts of data are collected, containing hidden information that can be utilized to make effective predictions and informed decisions.
By employing advanced data mining techniques, such as neural networks, we can develop heart disease prediction systems that use various medical parameters, including age, sex, blood pressure, cholesterol, and obesity, to estimate the risk level of heart disease. These predictive models provide significant knowledge and insights into the relationships between medical factors and patterns associated with heart disease.
- Data mining techniques play a crucial role in predicting heart disease.
- Advanced algorithms, such as neural networks, improve the accuracy of predictive models.
- Data mining enables healthcare practitioners to make informed clinical decisions.
- Predictive models provide insights into the relationships between medical factors and heart disease.
- By analyzing large datasets, data mining helps identify the risk level of heart disease.
Importance of Data Mining in Heart Disease Prediction
When it comes to predicting heart disease, data mining shines as a powerful tool. Its ability to extract hidden patterns and insights from large datasets proves invaluable in the healthcare industry. By analyzing and processing collected data, data mining techniques can identify relevant factors contributing to the occurrence of heart disease.
With the derived information, accurate predictive models can be developed, enabling healthcare practitioners to make more informed clinical decisions. But what exactly makes data mining so important in heart disease prediction?
- Improved Prognostic Accuracy: Data mining aids in enhancing the accuracy of heart disease prediction models. By analyzing vast amounts of data, it can identify complex relationships and patterns that may not be apparent through traditional statistical methods. This allows for more precise risk assessment and prognosis.
- Early Detection of At-Risk Individuals: Data mining enables the identification of individuals who are at high risk of developing heart disease. By analyzing various medical parameters, such as age, sex, blood pressure, cholesterol, and obesity, data mining can identify patterns that indicate a higher likelihood of developing the condition. This early detection allows for timely intervention and preventive measures.
- Personalized Treatment Strategies: Data mining aids in the development of personalized treatment strategies for heart disease patients. By analyzing the data collected from individuals with similar risk profiles and treatment outcomes, data mining can provide insights into the most effective treatments for different patient groups. This individualized approach improves patient outcomes and reduces healthcare costs.
Data mining’s contribution to heart disease prediction encompasses significant benefits that have the potential to revolutionize healthcare. Its ability to extract hidden patterns, improve prognostic accuracy, enable early detection, and facilitate personalized treatment strategies make it an indispensable tool in the fight against heart disease.
Data Mining Techniques for Heart Disease Prediction
In the prediction of heart disease, various data mining techniques are employed to extract valuable insights from large datasets. One commonly used technique is the application of neural networks, specifically the multilayer perceptron neural network with the backpropagation algorithm. This approach involves creating a structured database system that utilizes medical parameters such as age, sex, chest pain type, and cholesterol levels to train the neural network.
By analyzing the collected data and applying the appropriate algorithms, these data mining techniques can accurately predict the risk level of heart disease. The multilayer perceptron neural network, with its ability to learn complex patterns and relationships, plays a crucial role in capturing the intricate interplay between different medical factors.
With data mining techniques, healthcare professionals can gain valuable insights into the underlying patterns associated with heart disease. These predictive models enable early detection of individuals at risk, allowing for timely intervention and personalized treatment strategies to mitigate the progression of the disease.
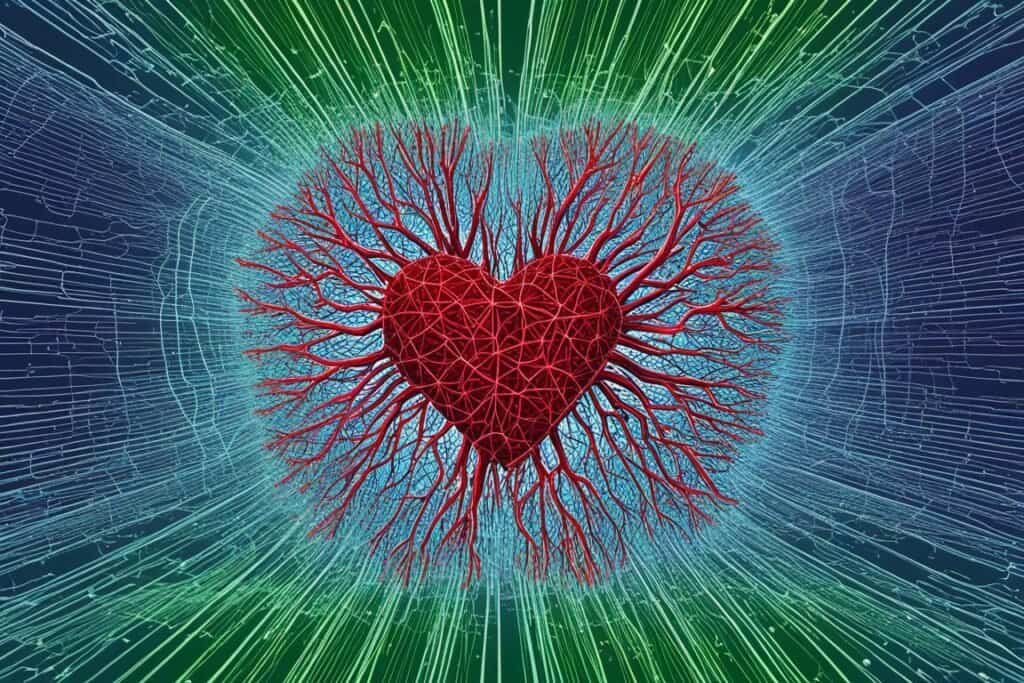
Implementing data mining techniques enables informed decision-making by healthcare practitioners, leading to improved patient outcomes and a more efficient healthcare system. The integration of such predictive models into clinical practice can enhance risk assessment, optimize treatment plans, and contribute to the overall management of heart disease.
Benefits of Data Mining in Heart Disease Prognosis
Data mining techniques offer several benefits in the prediction and prognosis of heart disease. By leveraging these techniques, healthcare practitioners can extract valuable insights and patterns from large datasets, leading to improved diagnosis and treatment strategies. Here are the key benefits of utilizing data mining in heart disease prognosis:
- Identification of High-Risk Patients: Data mining allows for the identification of individuals who are at a higher risk of developing heart disease. By analyzing a range of medical parameters such as age, sex, blood pressure, cholesterol levels, and more, data mining algorithms can identify patterns that indicate a higher likelihood of heart disease. This enables healthcare professionals to target preventive strategies and interventions for those individuals, potentially reducing the risk and improving their overall health outcomes.
- Personalized Treatment Plans: Data mining techniques can assist in the development of personalized treatment plans for heart disease patients. By analyzing patient data from various sources, including medical history, lifestyle factors, genetic information, and treatment outcomes, data mining algorithms can uncover patterns that suggest the most effective treatment approaches for individual patients. This individualized approach can lead to improved patient outcomes, as treatments can be tailored to each patient’s unique characteristics and needs.
- Improved Accuracy of Heart Disease Prediction: Data mining algorithms have the potential to improve the accuracy of heart disease prediction. By analyzing large datasets and identifying hidden patterns, these algorithms can make more accurate predictions about an individual’s likelihood of developing heart disease. This can help healthcare practitioners in early detection, intervention, and prevention strategies, ultimately leading to better health outcomes for patients.
- Cost-Effective Healthcare: Implementing data mining techniques in heart disease prognosis can result in cost savings for healthcare organizations. By accurately identifying high-risk patients and implementing preventive measures, healthcare providers can potentially reduce the need for expensive procedures and treatments related to advanced stages of heart disease. This can lead to cost savings for both patients and healthcare systems, making quality healthcare more accessible and affordable.
By harnessing the power of data mining, healthcare practitioners can unlock valuable insights that aid in the prediction and prognosis of heart disease. These insights enable the development of personalized treatment plans, improve accuracy in predicting heart disease, and contribute to cost-effective healthcare. With further advancements and research in data mining techniques, the benefits to heart disease prognosis are expected to continue growing, leading to improved patient outcomes and better healthcare strategies.
Challenges in Heart Disease Prediction using Data Mining
While data mining techniques have proven to be effective in heart disease prediction, there are several challenges that need to be addressed. One of the main challenges is the availability of comprehensive and accurate datasets. The quality of the collected data greatly affects the accuracy of the predictive models. Without high-quality data, the predictive accuracy may be compromised and result in unreliable predictions.
Another challenge in heart disease prediction using data mining is the need for advanced algorithms and computational resources to handle large datasets. As the volume of healthcare data continues to increase exponentially, traditional algorithms may struggle to analyze and extract meaningful insights from such vast amounts of information. Developing and implementing more sophisticated data mining algorithms is essential to ensure the accurate prediction of heart disease.
Additionally, the interpretability and transparency of the predictive models also pose challenges. Complex data mining algorithms can make accurate predictions, but they may not provide clear explanations for their decisions. The lack of interpretability can hinder healthcare professionals’ trust in the predictive models and limit their adoption in clinical practice. Ensuring transparency and interpretability in data mining algorithms is crucial to gain the confidence of healthcare practitioners.
Addressing these challenges is vital to improving the accuracy and reliability of heart disease prediction using data mining techniques. By overcoming these issues, healthcare professionals can harness the full potential of data mining to develop effective predictive models that aid in early detection and personalized treatment strategies for heart disease patients.

Future Directions in Data Mining for Heart Disease Prediction
The field of data mining for heart disease prediction is constantly evolving, and there are several future directions and advancements that hold promise. One area of focus is the integration of other advanced technologies, such as artificial intelligence and machine learning, to enhance the accuracy and efficiency of predictive models. By leveraging these technologies, healthcare practitioners can further improve their ability to predict heart disease and provide timely interventions.
In addition, the incorporation of genetic and genomic data into the predictive models can provide a more comprehensive understanding of an individual’s risk for heart disease. Genetic factors can play a significant role in determining an individual’s susceptibility to heart disease. By analyzing genetic data alongside traditional medical parameters, such as age, sex, and cholesterol levels, data mining techniques can uncover important genetic markers that contribute to heart disease risk. This integration of genetic and genomic data has the potential to revolutionize heart disease prediction and enable more personalized and targeted interventions.
Furthermore, the development of user-friendly and accessible data mining tools is crucial for the widespread adoption of these techniques in clinical practice. Currently, data mining algorithms can be complex and require technical expertise to implement. However, by designing intuitive interfaces and providing clear documentation, healthcare practitioners can easily incorporate data mining techniques into their daily workflow, improving patient care and outcomes.
Advancements in Data Mining Techniques for Heart Disease Prognosis
Advancements | Benefits |
---|---|
Integration of artificial intelligence and machine learning | Enhanced accuracy and efficiency of predictive models |
Incorporation of genetic and genomic data | Comprehensive understanding of an individual’s heart disease risk |
Development of user-friendly data mining tools | Widespread adoption and integration of data mining techniques into clinical practice |
The future of data mining for heart disease prediction is promising. By embracing advancements in technology and incorporating genetic and genomic data, healthcare practitioners can further improve the accuracy of predictive models and personalize interventions. Moreover, the development of user-friendly data mining tools will empower healthcare professionals to harness the full potential of data mining techniques in the prevention and management of heart disease.
Conclusion
Data mining techniques have revolutionized the prediction of heart disease by enabling the extraction of hidden patterns and insights from large datasets. Through the use of advanced algorithms, such as neural networks, the accuracy of predictive models has greatly improved. This allows healthcare practitioners to make informed clinical decisions based on reliable predictions. Despite the challenges in implementing data mining techniques, the benefits of accurate heart disease prediction and personalized treatment strategies far outweigh any limitations.
As the field of data mining continues to advance, future research should focus on integrating other technologies and expanding the scope of predictors. By incorporating artificial intelligence and machine learning, we can enhance the accuracy and efficiency of predictive models for heart disease. Additionally, the inclusion of genetic and genomic data can provide a more comprehensive understanding of an individual’s risk for heart disease.
The development of user-friendly and accessible data mining tools is also essential for promoting widespread adoption and integration of these techniques into clinical practice. By simplifying the process and making it more user-friendly, healthcare practitioners can easily apply data mining in the prediction and prognosis of heart disease. This will ultimately lead to better healthcare outcomes for patients.
In conclusion, data mining techniques have revolutionized the prediction of heart disease, enabling healthcare practitioners to extract valuable insights from large datasets. The use of advanced algorithms and the integration of other technologies hold promise for further enhancing the accuracy and effectiveness of predictive models. By leveraging data mining techniques, we can continue to improve heart disease prediction and contribute to personalized treatment strategies.